Medical imaging is a posh discipline the place decoding outcomes might be difficult.
AI fashions can help medical doctors by analyzing pictures that may point out disease-indicating anomalies.
Nonetheless, there’s a catch: these AI fashions normally provide you with a single answer when, in actuality, medical pictures typically have a number of interpretations.
In case you ask 5 consultants to stipulate an space of curiosity, like a small lump in a lung scan, you would possibly find yourself with 5 totally different drawings, as they may all have their very own opinions on the place the lump begins and ends, for instance.
To deal with this drawback, researchers from MIT, the Broad Institute of MIT Harvard, and Massachusetts Common Hospital have created Tyche, an AI system that embraces the paradox in medical picture segmentation.
Segmentation includes labeling particular pixels in a medical picture that symbolize necessary buildings, like organs or cells.
Marianne Rakic, an MIT pc science PhD candidate and lead creator of the research, explains, “Having choices will help in decision-making. Even simply seeing that there’s uncertainty in a medical picture can affect somebody’s selections, so it is very important take this uncertainty into consideration.”
Named after the Greek goddess of likelihood, Tyche generates a number of attainable segmentations for a single medical picture to seize ambiguity.
Every segmentation highlights barely totally different areas, permitting customers to decide on probably the most appropriate one for his or her wants.
Rakic tells MIT Information, “Outputting a number of candidates and guaranteeing they’re totally different from each other actually offers you an edge.”
So, how does Tyche work? Let’s break it down into 4 easy steps:
- Studying by instance: Customers give Tyche a small set of instance pictures, known as a “context set,” that present the segmentation process they need to carry out. These examples can embrace pictures segmented by totally different human consultants, serving to the mannequin perceive the duty and the potential for ambiguity.
- Neural community tweaks: The researchers modified a normal neural community structure to permit Tyche to deal with uncertainty. They adjusted the community’s layers in order that the potential segmentations generated at every step may “talk” with one another and the context set examples.
- A number of potentialities: Tyche is designed to output a number of predictions based mostly on a single medical picture enter and the context set.
- Rewarding high quality: The coaching course of was tweaked to reward Tyche for producing the very best prediction. If the consumer asks for 5 predictions, they will see all 5 medical picture segmentations produced by Tyche, even when one is perhaps higher.
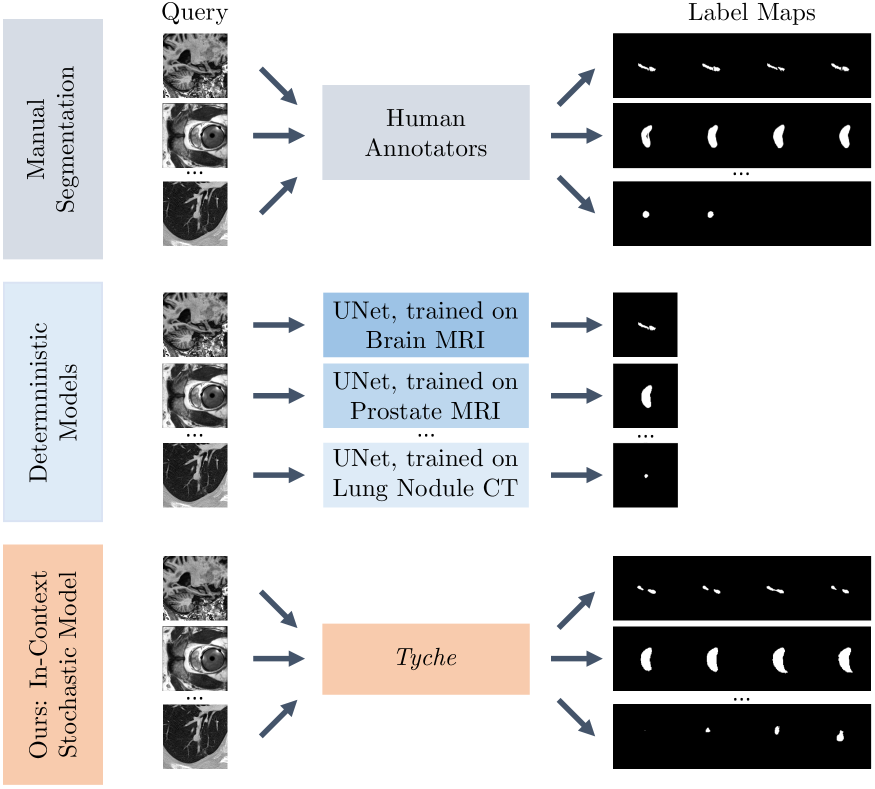
One in every of Tyche’s largest strengths is its adaptability. It could actually tackle new segmentation duties while not having to be retrained from scratch.
Usually, AI fashions for medical picture segmentation use neural networks that require in depth coaching on giant datasets and machine studying experience.
In distinction, Tyche can be utilized “out of the field” for varied duties, from recognizing lung lesions in X-rays to figuring out mind abnormalities in MRIs.
Quite a few research have been performed in AI medical imaging, together with main breakthroughs in breast most cancers screening and AI diagnostics that match and even beat medical doctors in decoding pictures.
Trying to the long run, the analysis workforce plans to discover utilizing extra versatile context units, presumably together with textual content or a number of varieties of pictures.
Additionally they need to develop methods to enhance Tyche’s worst predictions and allow the system to advocate one of the best segmentation candidates.